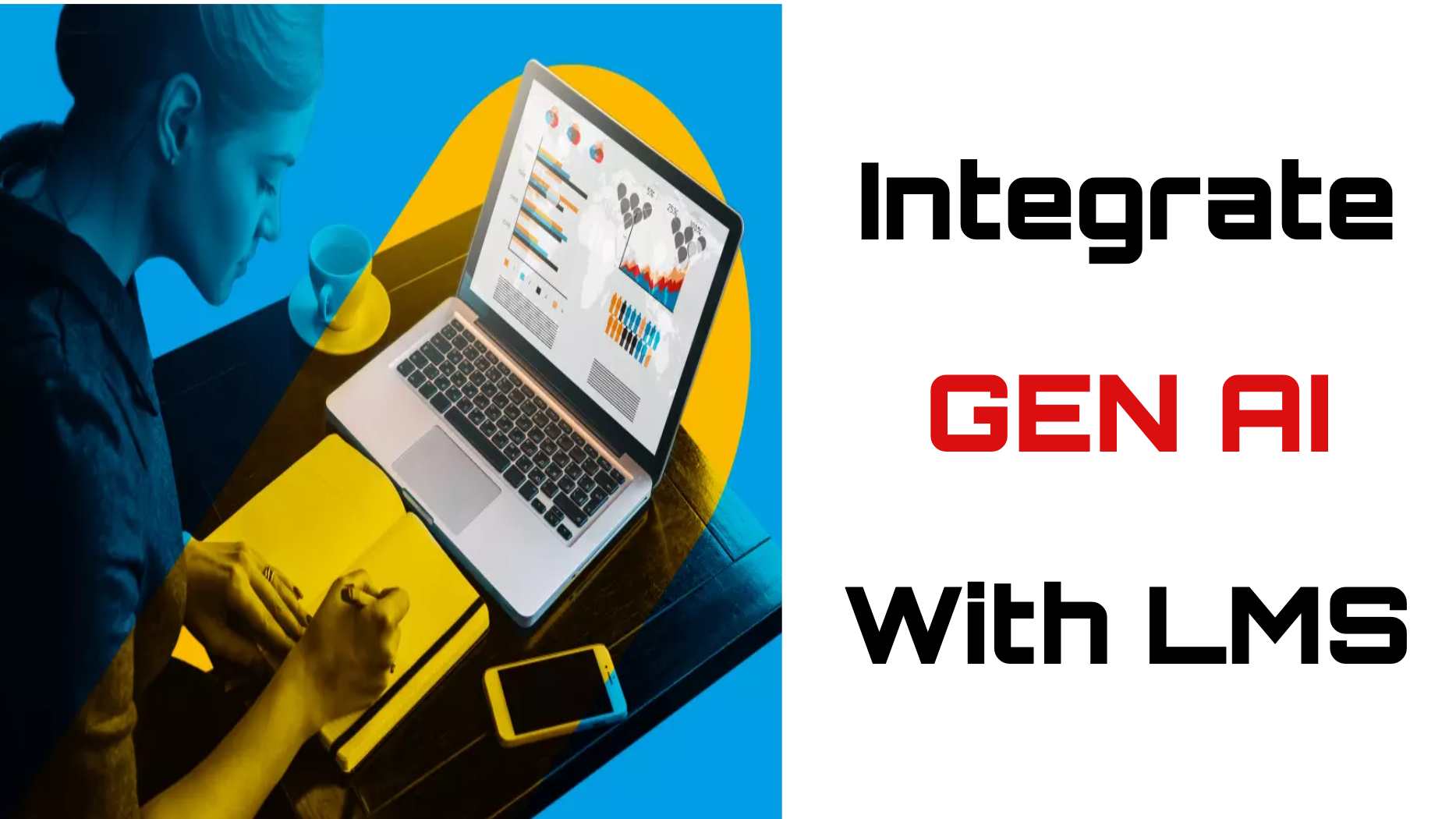
Understanding Generative AI
Generative AI is a subset of artificial intelligence (AI) focused on creating new content rather than simply analyzing existing data or making decisions based on predefined rules. Unlike other forms of AI, such as machine learning algorithms used for classification or prediction tasks, Generative AI is specifically designed to generate new content that resembles human-created data.
At its core, Generative AI works by learning patterns and structures from large datasets, often using neural networks or other advanced algorithms. These models are trained on vast amounts of data, such as text, images, or videos, to learn the underlying patterns and relationships within the data.
Once trained, Generative AI can then generate new content that resembles the input data it was trained on. For example:
- In the case of text generation, a Generative AI model can produce coherent paragraphs of text that mimic the style and vocabulary of the training text.
- For image generation, Generative AI can create realistic images that resemble the patterns and features found in the training images.
- Similarly, in video generation, Generative AI can produce new video sequences by learning the temporal and spatial relationships between frames in the training videos.
What sets Generative AI apart from other forms of AI is its ability to create content that is not explicitly present in the training data. Instead, it learns the underlying distribution of the data and uses that knowledge to generate new, novel content that aligns with the learned patterns.
However, it's important to note that while Generative AI can create remarkably realistic content, it is not without limitations. Generated content may sometimes exhibit inconsistencies or errors, especially when pushed beyond the boundaries of the training data. Additionally, ensuring that generated content is accurate, ethical, and appropriate for its intended use requires careful oversight and validation by humans.
Benefits of Integrating Generative AI into an LMS
-
Personalized Learning: Generative AI enables personalized learning experiences by tailoring content to individual students' needs and preferences. This customization can encompass various aspects, including:
- Custom Learning Materials: Generative AI can create learning materials such as tutorials, exercises, and study guides tailored to students' learning styles, interests, and proficiency levels.
- Adaptive Content Delivery: By analyzing students' performance and preferences, Generative AI can dynamically adjust the presentation and difficulty of learning materials to optimize engagement and comprehension.
- Individualized Feedback: Generative AI can generate personalized feedback on assignments and assessments, pinpointing areas for improvement and offering targeted suggestions for further learning.
-
Content Creation: Generative AI empowers educators to create diverse and engaging learning resources efficiently. Key advantages include:
- Automated Resource Generation: Generative AI can assist educators in generating a wide range of learning materials, including quizzes, interactive simulations, and multimedia presentations.
- Rapid Iteration: With Generative AI, educators can quickly iterate and refine learning resources based on feedback and changing educational needs, streamlining the content creation process.
- Augmented Creativity: Generative AI can inspire educators by generating novel ideas and perspectives, sparking creativity and innovation in curriculum development.
-
Feedback and Assessment: Generative AI streamlines the feedback and assessment process, benefiting both educators and students:
- Automated Assessment: Generative AI can automatically evaluate student submissions, providing instant feedback on correctness, completeness, and conceptual understanding.
- Consistent Evaluation: Generative AI ensures consistency in grading standards and feedback delivery, reducing bias and subjectivity in assessment.
- Time Savings: By automating routine grading tasks, Generative AI frees up educators' time to focus on more meaningful aspects of teaching, such as mentoring and individualized instruction.
-
Adaptive Learning Paths: Generative AI enhances adaptive learning by dynamically adjusting learning paths to optimize student outcomes:
- Personalized Recommendations: Generative AI analyzes students' learning histories and performance data to suggest tailored learning paths and supplementary resources.
- Real-time Adaptation: Generative AI continuously monitors students' progress and comprehension, adapting learning paths in real-time to address emerging needs and challenges.
- Mastery-based Learning: Generative AI promotes mastery-based learning by offering personalized pathways that prioritize mastery of core concepts before advancing to more complex topics, fostering deeper understanding and retention.
-
Accessibility: Generative AI promotes accessibility by facilitating the creation of inclusive learning materials:
- Alternative Formats: Generative AI can generate alternative formats of learning materials, such as audio descriptions for images, transcripts for videos, and tactile graphics for visually impaired students.
- Multimodal Learning: Generative AI enables the conversion of text-based content into audio or visual formats, catering to diverse learning preferences and abilities.
- Language Translation: Generative AI supports language translation and localization, making learning materials accessible to students from different linguistic backgrounds and cultures.
Use Cases
-
Automatically generating quiz questions and answers based on course content:
- In an LMS, Generative AI can analyze the course material and automatically generate quiz questions of varying difficulty levels, covering different topics and concepts.
- The AI can also generate corresponding answer choices, ensuring that questions are well-constructed and aligned with learning objectives.
- By automating the quiz creation process, educators can save time and ensure that assessments are consistently relevant and challenging.
-
Creating interactive simulations or virtual labs to enhance hands-on learning experiences:
- Generative AI can be used to create interactive simulations or virtual labs that allow students to explore complex concepts in a hands-on, immersive manner.
- For example, in a science course, Generative AI can generate virtual experiments where students can manipulate variables and observe real-time outcomes.
- These simulations can provide valuable opportunities for experimentation and discovery, enhancing students' understanding and retention of course material.
-
Generating summaries or explanations of complex concepts to aid student comprehension:
- Generative AI can analyze course content and generate summaries or explanations of complex concepts in a concise and easy-to-understand format.
- These summaries can serve as supplementary learning materials, helping students review key concepts and reinforce their understanding.
- Additionally, Generative AI can generate personalized explanations tailored to individual students' learning preferences and comprehension levels.
-
Personalizing learning paths based on students' interests, learning styles, and performance:
- Generative AI can analyze students' learning profiles, including their interests, learning styles, and performance data, to personalize learning paths.
- For example, the AI can recommend additional resources, activities, or learning modules based on students' unique needs and preferences.
- By personalizing learning paths, Generative AI can optimize student engagement, motivation, and learning outcomes, catering to each student's individual strengths and challenges.
Challenges and Considerations
-
Ethical concerns:
- Bias in generated content: Generative AI models can inadvertently perpetuate biases present in the training data, leading to biased or unfair content generation. It's essential to carefully curate training data and implement bias mitigation strategies to minimize such risks.
- Privacy concerns: Generative AI may require access to sensitive student data to personalize learning experiences, raising concerns about data privacy and security. Strict privacy policies and robust data protection measures should be implemented to safeguard student information.
-
Quality control:
- Accuracy and relevance of generated content: Despite advancements in Generative AI, there may still be instances of inaccuracies or irrelevant content generation. Human oversight and validation are essential to ensure the quality and relevance of generated materials, particularly in educational contexts where accuracy is paramount.
- Validation processes: Establishing rigorous validation processes, including peer review and expert evaluation, can help identify and rectify any inaccuracies or inconsistencies in generated content before it is deployed within the LMS.
-
Integration complexity:
- Technical requirements: Integrating Generative AI into existing LMS platforms may require significant technical expertise and resources. It's essential to assess the technical infrastructure and capabilities of the LMS to ensure compatibility with Generative AI solutions.
- Compatibility issues: Generative AI models may need to be adapted or customized to align with the specific requirements and functionalities of the LMS. Compatibility testing and collaboration between AI developers and LMS providers are necessary to address any compatibility issues effectively.
- Training data availability: Generative AI models rely on large amounts of high-quality training data to generate accurate and relevant content. Access to sufficient and diverse training data, including educational resources and student performance data, may pose challenges in certain educational contexts. Collaboration with educational institutions and data sharing initiatives can help address data availability constraints.
By proactively addressing these challenges and considerations, educators and technology developers can mitigate risks and maximize the benefits of integrating Generative AI into LMS platforms, ultimately enhancing teaching and learning experiences.
Best Practices for Implementation
-
Start small:
-
Begin with pilot projects or small-scale implementations to test the capabilities and effectiveness of Generative AI within your LMS. Starting small allows you to assess the feasibility and impact of the technology without committing extensive resources upfront.
- Choose specific use cases or scenarios where Generative AI can address tangible educational challenges or enhance existing processes. This targeted approach enables focused experimentation and evaluation of the technology's benefits and limitations.
-
-
Collaborate with stakeholders:
- Involve educators, students, administrators, and IT professionals from the outset of the implementation process. Collaborating with stakeholders ensures that the Generative AI solution is designed and tailored to meet the diverse needs and preferences of all user groups.
- Solicit feedback and input from stakeholders throughout the implementation process to identify key requirements, preferences, and concerns. Engaging stakeholders as active participants fosters a sense of ownership and investment in the success of the Generative AI integration.
-
Continuous improvement:
- Adopt an iterative approach to implementation and refinement, focusing on ongoing evaluation and optimization of the Generative AI integration.
- Establish mechanisms for collecting and analyzing feedback from users, including educators and students, to identify areas for improvement and enhancement.
- Monitor key performance metrics, such as student engagement, learning outcomes, and user satisfaction, to assess the impact of Generative AI on teaching and learning effectiveness.
- Use insights gained from feedback and performance metrics to iterate on the Generative AI solution, addressing identified issues and incorporating new features or enhancements as needed.
By following these best practices, educators and educational institutions can effectively integrate Generative AI into their LMS platforms, maximizing the benefits of the technology and enhancing teaching and learning experiences for students.
How TechSolvo Can Help Integrate Generative AI Inside Your Existing LMS
-
Customized Solutions: We work closely with educational institutions to understand their specific requirements and design customized Generative AI solutions that align with their goals and objectives.
-
Technical Expertise: Our team of AI engineers and developers possesses advanced technical skills and expertise in implementing Generative AI algorithms and models within LMS environments.
-
Collaboration and Support: We collaborate closely with educators, administrators, and IT professionals throughout the implementation process, ensuring that the Generative AI integration meets the needs and expectations of all stakeholders.
-
Training and Support: We provide comprehensive training and support to educators and LMS administrators to ensure they are proficient in using Generative AI tools and features effectively.
-
Continuous Improvement: We emphasize a culture of continuous improvement, regularly monitoring and evaluating the Generative AI integration to identify areas for enhancement and optimization based on user feedback and performance metrics.
With TechSolvo's expertise and support, educational institutions can harness the power of Generative AI to enhance teaching and learning experiences, drive student engagement and success, and achieve their educational objectives effectively. Contact us today to learn more about how we can help integrate Generative AI into your existing LMS
Insights
To properly understand the things that are prevalent in the industries, keeping up-to-date with the news is crucial. Take a look at some of our expertly created blogs, based on full-scale research and statistics on current market conditions.
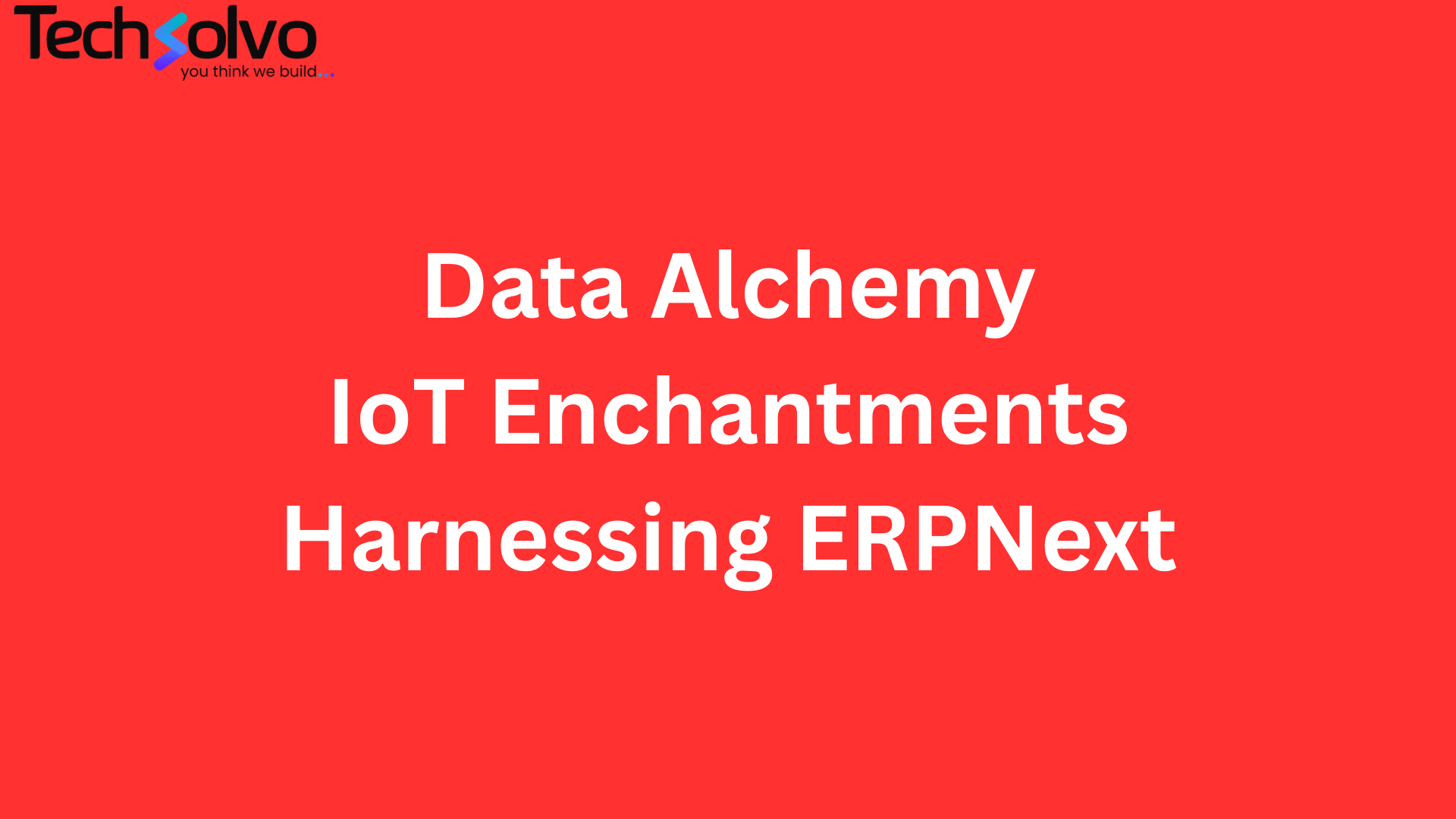
Unlocking the Power of Data Analytics for IoT Devices with ERPNext
Explore how businesses can gain real-time insights from IoT devices using modern data ana…
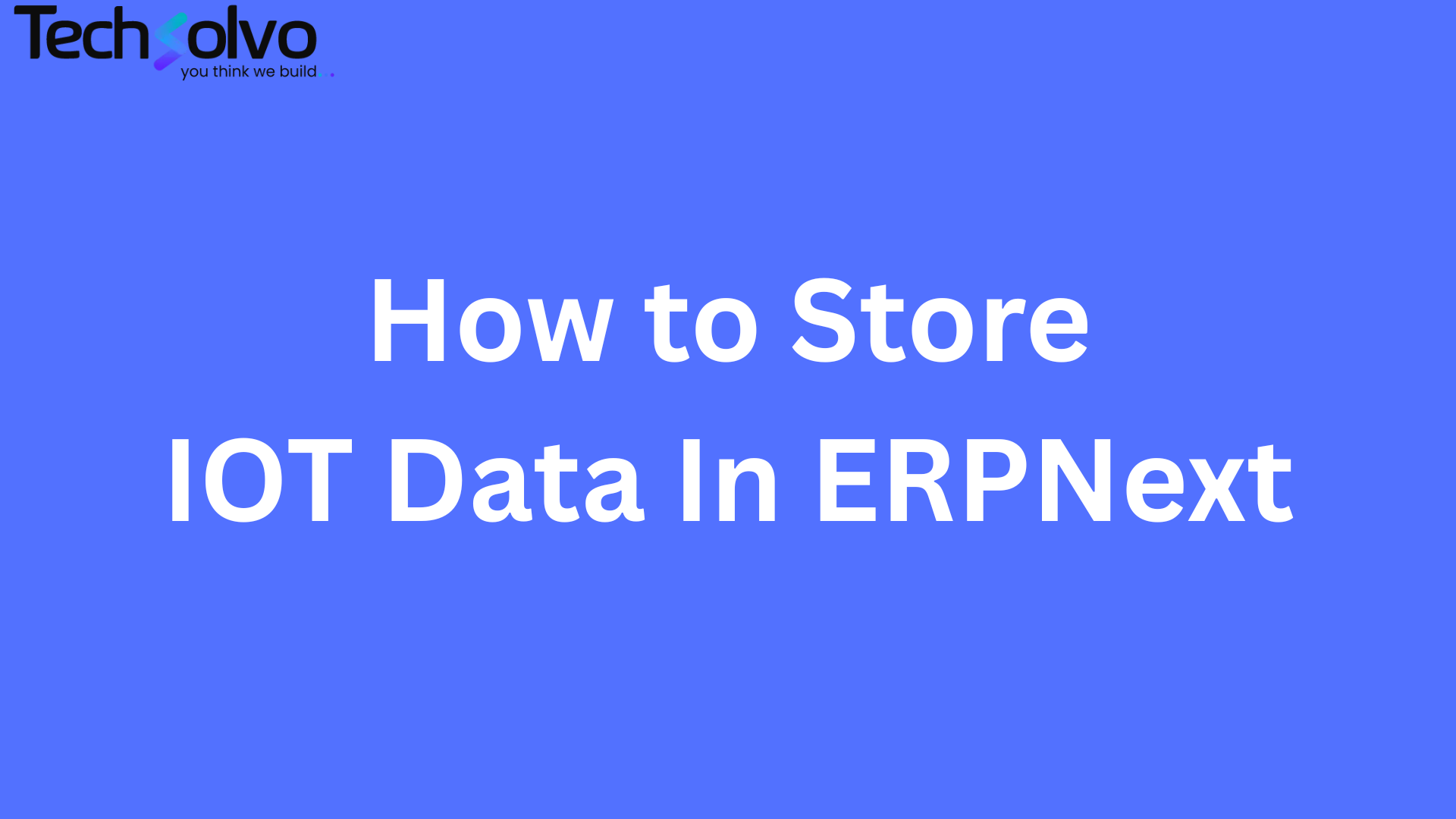
How to Store IoT Data Inside ERPNext: A Complete Guide for IoT Companies
A complete guide for IoT and manufacturing companies on integrating machine-generated dat…
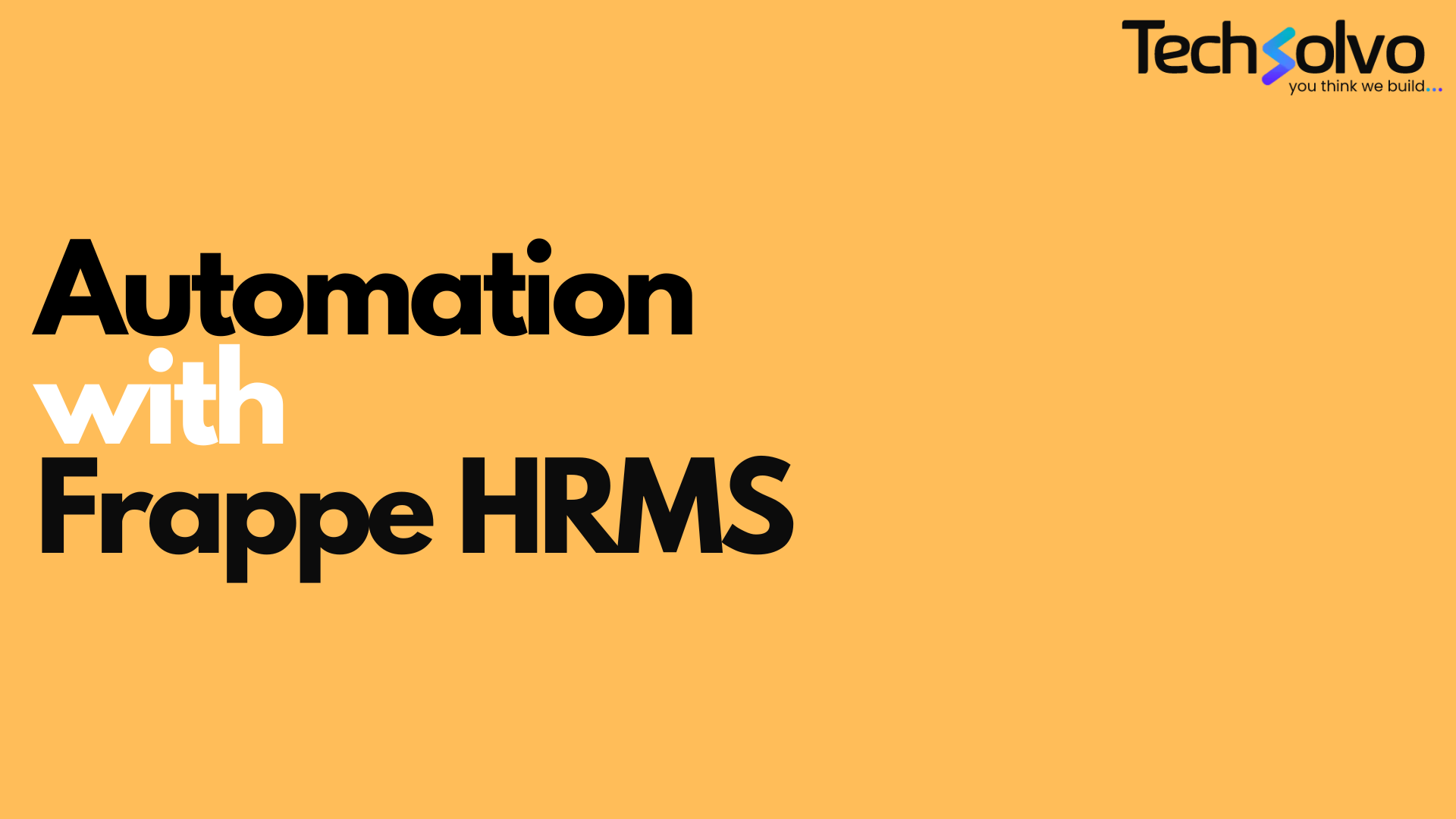
Automation in Human Resource Management with Frappe HRMS
Discover how HR automation with Frappe HRMS can streamline HR operations, enhance employe…