10 Ways Techsolvo Helps Businesses in Predictive Analytics:
-
Uncover Hidden Patterns and Trends: Techsolvo's advanced analytics tools go beyond basic data analysis to reveal hidden patterns and relationships within your data, enabling you to make informed decisions based on real-time insights.
-
Predict Future Outcomes with Accuracy: Leverage Techsolvo's machine learning expertise to build powerful predictive models that forecast future trends and outcomes with remarkable accuracy, allowing you to proactively prepare for any eventuality.
-
Minimize Risks and Maximize Opportunities: Techsolvo's risk assessment and opportunity identification solutions help you anticipate and mitigate potential risks while capitalizing on emerging opportunities, ensuring your business stays ahead of the curve.
-
Optimize Resource Allocation: Make data-driven decisions about resource allocation with Techsolvo's resource optimization tools, ensuring your personnel, inventory, and budget are directed towards areas with the highest potential return.
-
Personalize Customer Experiences: Deliver hyper-personalized customer experiences using Techsolvo's advanced customer segmentation and targeting capabilities, resulting in increased customer satisfaction and loyalty.
-
Improve Operational Efficiency: Streamline your operations and identify areas for improvement with Techsolvo's operational efficiency solutions, leading to cost reductions and increased productivity.
-
Gain a Competitive Advantage: Stay ahead of your competition by leveraging Techsolvo's predictive analytics solutions to gain valuable insights into market trends and customer behavior, enabling you to develop winning strategies.
-
Make Data-Driven Decisions: Eliminate guesswork and intuition from your decision-making process with Techsolvo's data-driven insights, ensuring every decision is backed by concrete evidence and analysis.
-
Scale Your Business with Confidence: Techsolvo's scalable predictive analytics solutions can grow alongside your business, providing you with the insights and tools you need to succeed at every stage of your growth journey.
-
Boost Your Bottom Line: Ultimately, Techsolvo's predictive analytics solutions help you drive tangible business results, leading to increased revenue, improved profitability, and sustained growth.
Predictive Analytics Platforms
Frequently Asked Questions
Data analysis explores past trends and patterns, while predictive analytics uses those insights to forecast future outcomes. Imagine finding hidden connections in past sales data to predict customer churn or future demand.
Popular models include regression, which predicts continuous values like sales amounts, and classification, which categorizes data like customer churn risk. More advanced models like neural networks handle complex relationships between variables.
Quality data is key! You need relevant, accurate, and sufficient data related to your prediction goals. Historical sales data, customer demographics, market trends, and other factors might be necessary.
Accuracy depends on various factors like data quality, model choice, and complexity. Generally, expect estimates rather than absolute predictions. Model testing and validation are crucial to assess reliability.
Informed decision-making, optimized resource allocation, improved customer service, and identifying potential risks are just some advantages. Predictive analytics can be a powerful tool to gain a competitive edge.
Absolutely! Azure Machine Learning seamlessly integrates with various Microsoft and third-party tools, including Power BI for data visualization and Dynamics 365 for real-time insights.
Yes! Azure provides user-friendly interfaces and drag-and-drop features alongside coding options, making it accessible to both data scientists and business analysts.
From customer churn prediction and fraud detection to product recommendation and demand forecasting, Azure Machine Learning tackles a wide range of predictive tasks across various industries.
Scalability, affordability, and security are key benefits. You pay only for what you use, and the cloud infrastructure scales seamlessly to meet your needs. Plus, built-in security features protect your data and models.
It's a cloud-based platform offering tools and services to build, train, deploy, and manage machine learning models for predictive analytics tasks, eliminating the need for extensive coding.
AWS SageMaker is a fully managed service for building, training, and deploying machine learning models. It provides a complete set of tools and infrastructure to streamline the entire machine learning lifecycle, from data preparation to model deployment and monitoring.
SageMaker supports a wide variety of machine learning algorithms, including linear regression, classification, clustering, and deep learning.
SageMaker makes it easy to deploy your models as web services or batch transformations. You can also use SageMaker to create real-time inference applications.
SageMaker provides a variety of tools for monitoring your models, including metrics, logs, and visualizations. This helps you ensure that your models are performing well and meeting your business objectives.
SageMaker is available in all AWS regions. You can get started by creating a free AWS account and exploring the SageMaker console or SDKs.
From customer churn to equipment failure, the possibilities are vast. Use pre-built AI/ML models or build your own using Vantage's built-in tools. Explore 80+ use cases across various industries.
It's a managed platform offering services for the entire predictive analytics lifecycle, from data ingestion to model training and deployment. You get tools like Vertex AI Workbench, BigQuery ML, and AI Platform Pipelines, all in one place.
Yes, it caters to both beginners and experts. The Workbench provides a visual interface for building and deploying models, while advanced users can leverage pre-built AI services and coding flexibility.
You can build various models, including regressions, classifications, and anomaly detection, for tasks like forecasting, churn prediction, and fraudulent transaction identification.
Highly scalable. You can effortlessly adjust resources based on your needs, paying only for what you use. This makes it cost-effective for small and large projects alike.
Absolutely! Google Cloud offers a free tier with limited resources to explore the platform and learn the ropes before committing.
You'll build custom applications within Foundry, extending its analytical power to solve specific problems. Think creating dashboards, integrating data sources, and developing micro-models for predictive insights.
Strong Python, SQL, and data modeling skills are essential. Familiarity with APIs, cloud platforms, and machine learning is a plus. Bonus points for understanding Palantir's ontology and application development framework.
Diverse! From optimizing supply chains to predicting financial fraud, you'll tackle real-world challenges across industries like healthcare, government, and finance.
Foundry has a unique framework, so expect an initial learning phase. Palantir offers extensive training resources and a supportive community to help you navigate.
Palantir is rapidly growing, and skilled Foundry developers are in high demand. As AI and predictive analytics become increasingly crucial, you'll be at the forefront of shaping the future.
Viya is cloud-native, built for scale and collaboration. It's faster, more flexible, and integrates seamlessly with other tools. Think modern analytics powerhouse!
Strong statistics and programming (Python, R, SAS) are key. But it's not just about code; communication, problem-solving, and understanding business needs are crucial.
Not mandatory, but highly recommended. SAS certifications validate your skills and boost your resume. Start with SAS Certified Predictive Modeling Specialist or SAS Certified Data Miner.
They build and deploy predictive analytics solutions using SAS Viya, the platform powering next-gen analytics. Think machine learning models, AI-driven insights, and automated decision-making for businesses.
Absolutely! Predictive analytics is booming, and SAS Viya Developers are in high demand. Expect competitive salaries, exciting projects, and the chance to shape the future with data-driven decisions.
It's a cloud-based platform for developers to build and deploy predictive analytics pipelines on Teradata Vantage. Think streamlined SQL editing, API access, and easy integration with popular AI/ML tools like Python and R.
Popular IDEs like VS Code, Jupyter notebooks, and tools like Python, R, and Spark for distributed analytics. Seamless API access lets you extend Vantage with custom solutions.
If you're familiar with SQL and basic data science concepts, you're on the right track. Teradata offers extensive documentation, tutorials, and a free developer tier to experiment in.
Teradata's cloud platform boasts industry-leading security features and compliance certifications. You control access and encryption for your data and models.
Databricks is a cloud-based platform that unifies data lakehouse, data engineering, data science, and machine learning workflows. It provides a collaborative environment for teams to work on predictive analytics projects from data ingestion to model deployment.
Yes, Databricks integrates seamlessly with various data sources, BI tools, and ML libraries, allowing you to leverage your existing investments.
Databricks offers robust security features like encryption, access control, and audit trails to ensure data privacy and compliance.
Databricks pricing depends on your usage and required features. They offer flexible plans for startups, enterprises, and individual users.
Databricks provides comprehensive documentation, tutorials, and training courses to help you get started and master the platform.
It's a powerful data science platform offering visual and coding tools for building and deploying predictive models. Think drag-and-drop meets Python scripting for in-depth analysis.
From data preparation and wrangling to model building, evaluation, and deployment, RapidMiner Developer handles the entire predictive analytics lifecycle. It excels in tasks like churn prediction, fraud detection, and customer segmentation.
RapidMiner boasts an intuitive interface, rich library of pre-built operators, and seamless integrations with various data sources and platforms. It's also known for its scalability and robustness, handling large datasets with ease.
While powerful, it may not be suitable for highly complex tasks requiring extreme computational power or custom algorithms not readily available.
RapidMiner offers a free personal edition with limited features, while paid subscriptions unlock advanced functionalities and larger data processing capabilities.
H2O.ai developers specialize in building and deploying machine learning models using H2O, an open-source distributed AI platform. They work on projects like fraud detection, churn prediction, and demand forecasting.
Strong proficiency in Python, statistical modeling, and machine learning algorithms is crucial. Familiarity with cloud platforms like AWS or Azure is an advantage. Excellent communication and teamwork skills are also essential.
Absolutely! The demand for H2O.ai developers is booming across industries like finance, healthcare, and retail. As companies increasingly rely on AI, H2O.ai's ease of use and scalability make it a popular choice.
H2O.ai developers can progress to senior developer roles, lead data science teams, or even become AI architects. Opportunities to specialize in areas like natural language processing or computer vision are also available.
H2O.ai offers extensive online documentation, tutorials, and certification programs. Several universities also offer courses and bootcamps focused on H2O.ai and predictive analytics.
Beyond core TensorFlow knowledge, expertise in Autoencoders, Variational Autoencoders (VAEs), Generative Adversarial Networks (GANs), and Transformers is essential. Familiarity with libraries like TensorFlow Probability and TensorFlow Datasets is also valuable.
Building your own GAN for image or text generation, creating a VAE for data compression or anomaly detection, or implementing a Transformer-based language model demonstrate your understanding and practical application of these techniques.
Training Generative AI models can be computationally expensive and require careful hyperparameter tuning. Additionally, issues like bias and interpretability need to be addressed responsibly.
Follow TensorFlow's official blog and research papers, participate in the TensorFlow community forums and meetups, and explore open-source projects like TensorFlow Hub for pre-trained generative models.
The demand for Generative AI skills is booming across various industries. You could work on developing AI-powered content creation tools, generating synthetic data for training other AI models, or even pushing the boundaries of creative AI research.
PyTorch's dynamic computational graph allows for rapid prototyping and experimentation, ideal for exploring various model architectures and fine-tuning hyperparameters in predictive tasks.
Absolutely! PyTorch's extensive ecosystem includes TorchHub, a platform brimming with pre-trained models for tasks like image classification, NLP, and time series forecasting, saving you time and effort.
Finance, healthcare, e-commerce, and manufacturing heavily rely on predictive analytics. Tech giants and startups across various sectors seek PyTorch expertise for cutting-edge solutions.
PyTorch's flexibility and dynamic graph computation might be preferred for research and rapid prototyping, while TensorFlow's production-ready features and larger community might be preferable for large-scale deployments.
Online courses like Coursera's "Practical Deep Learning for Coders" by fast.ai, books like "Deep Learning with PyTorch" by Francois Chollet, and active participation in online communities like PyTorch Forum and Stack Overflow are great starting points.
Scikit-learn shines in its extensive library of pre-built machine learning algorithms, from regression to classification to clustering. Its simple, concise syntax and well-documented functions make it beginner-friendly and efficient.
Absolutely! Scikit-learn is written in Python, so proficiency is crucial. But fear not, basic to intermediate Python skills are often sufficient for most projects.
Scikit-learn empowers developers to quickly prototype and deploy predictive models. Its built-in cross-validation tools ensure robust model performance, while its visualization capabilities aid in understanding model behavior.
Feature engineering and data pre-processing can be intricate, requiring domain expertise. Choosing the right algorithm for the task can be challenging, and hyperparameter tuning can be time-consuming.
Scikit-learn's continuous development ensures its relevance in the evolving field of machine learning. Integration with cutting-edge algorithms and improved scalability promise to empower developers in building even more sophisticated predictive models.
They build and deploy machine learning models for predictive analytics using Spark's MLlib library. This involves data wrangling, feature engineering, model training and evaluation, and integrating models into production systems.
Strong proficiency in Scala, Python, or both, along with a solid understanding of statistics, machine learning algorithms, and distributed computing concepts. Experience with Spark, MLlib, and related libraries like Spark SQL and TensorFlow is crucial.
Demand is high in various industries like finance, healthcare, retail, and tech. Roles include Machine Learning Engineer, Data Scientist, and Predictive Analytics Specialist.
Yes, it requires dedication and continuous learning. Resources like Apache Spark documentation, online courses, and communities provide a good starting point.
Continuous development focuses on improving scalability, performance, and integrating with emerging technologies like AI and deep learning.
Strong understanding of machine learning algorithms, proficiency in Python and related libraries (NumPy, Pandas, Scikit-learn), experience with data wrangling and feature engineering, and excellent analytical and problem-solving abilities are crucial.
XGBoost's versatility makes it popular across various sectors like finance, healthcare, e-commerce, and manufacturing for tasks like fraud detection, churn prediction, personalized recommendations, and anomaly detection.
The demand for XGBoost skills is rapidly growing, with attractive salaries and career advancement opportunities in data science, analytics, and AI fields.
Online courses, tutorials, documentation, and Kaggle competitions provide excellent learning opportunities. Additionally, contributing to open-source XGBoost projects can enhance your skills and portfolio.
Salary varies based on experience, location, and industry, but XGBoost skills generally command premium salaries compared to other developer roles.
They build and implement machine learning models, primarily Facebook's Prophet forecasting tool, to predict future business trends, optimize resource allocation, and drive data-driven decisions.
Strong Python and statistics knowledge, proficiency in Prophet and other forecasting libraries, data wrangling and analysis skills, and communication to translate technical insights into business value.
Advance as a senior Prophet developer, specialize in specific forecasting domains like finance or retail, transition into data science or machine learning engineering roles, or lead predictive analytics projects.
Dealing with messy or incomplete data, choosing the right forecasting model for the problem, interpreting model outputs for non-technical stakeholders, and continuously monitoring and improving model performance.
Yes! The demand for skilled Prophet developers is booming across industries, offering ample job opportunities, competitive salaries, and the chance to directly impact business outcomes through the power of predictive analytics.
Strong Python and machine learning fundamentals are crucial, along with proficiency in Keras libraries like TensorFlow and PyTorch. Familiarity with statistical analysis and data visualization tools is also valuable.
They build and deploy predictive models for various tasks, including demand forecasting, fraud detection, sentiment analysis, and image recognition.
They can specialize in specific domains like healthcare, finance, or marketing, or move into research, data science leadership, or even entrepreneurship.
While it has a gentle learning curve compared to other deep learning frameworks, it still requires dedication and practice. Online courses, tutorials, and communities can provide valuable support.
The demand for skilled Keras developers is expected to surge as organizations increasingly adopt AI and machine learning solutions. With continuous advancements in the field, staying updated and adaptable will be key to success.
Strong proficiency in R programming, statistical modeling, and data manipulation are crucial. Familiarity with machine learning algorithms, cloud platforms, and version control systems like Git is highly valued.
You'll collaborate with data scientists to develop and deploy predictive models, clean and analyze large datasets, build interactive dashboards, and automate repetitive tasks using R scripts.
You can advance to senior developer roles, specialize in specific areas like fraud detection or healthcare analytics, or transition into data science or machine learning engineering.
Package compatibility issues, limited debugging tools compared to Python, and scalability for handling massive datasets can be hurdles.
Following R blogs and communities, attending conferences, and participating in online hackathons are great ways to stay ahead of the curve.
Julia shines in rapid prototyping and high-performance analytics due to its speed, conciseness, and powerful statistical libraries. It seamlessly integrates with Python and R, leveraging existing data science ecosystems.
Julia excels in building complex models, parallel computing for faster training, and creating custom algorithms. Its multiple dispatch system enables efficient handling of diverse data types.
Julia's syntax feels familiar to those with Python or R background, making it easier to pick up. However, its functional programming core and metaprogramming features might require a paradigm shift.
Demand for Julia developers in predictive analytics is growing across industries like finance, healthcare, and tech. Companies value its efficiency and performance for building cutting-edge analytical solutions.
Numerous online resources like tutorials, courses, and communities cater to Julia learners. Additionally, books and documentation specifically geared towards predictive analytics with Julia are available.
It's a powerful open-source gradient boosting library like XGBoost, but specifically designed for categorical features. This means it handles non-numerical data elegantly, boosting performance and interpretability.
Unlike other Gradient Boosting libraries, CatBoost excels with categorical features, often leading to higher accuracy with less data manipulation. Its built-in model explainability tools also make understanding your model's reasoning much easier.
CatBoost offers both high-level APIs and fine-grained parameter tuning for experienced users. Its Python and R interfaces are intuitive, and plenty of tutorials and documentation are available to get you started.
Both are Gradient Boosting powerhouses, but CatBoost often shines with categorical features and interpretability. XGBoost might be a better choice for purely numerical data or large-scale distributed training.
While CatBoost handles categorical data beautifully, it can be slower than XGBoost for purely numerical datasets. Additionally, its community and resources are not as vast as XGBoost's.
Insights
To properly understand the things that are prevalent in the industries, keeping up-to-date with the news is crucial. Take a look at some of our expertly created blogs, based on full-scale research and statistics on current market conditions.
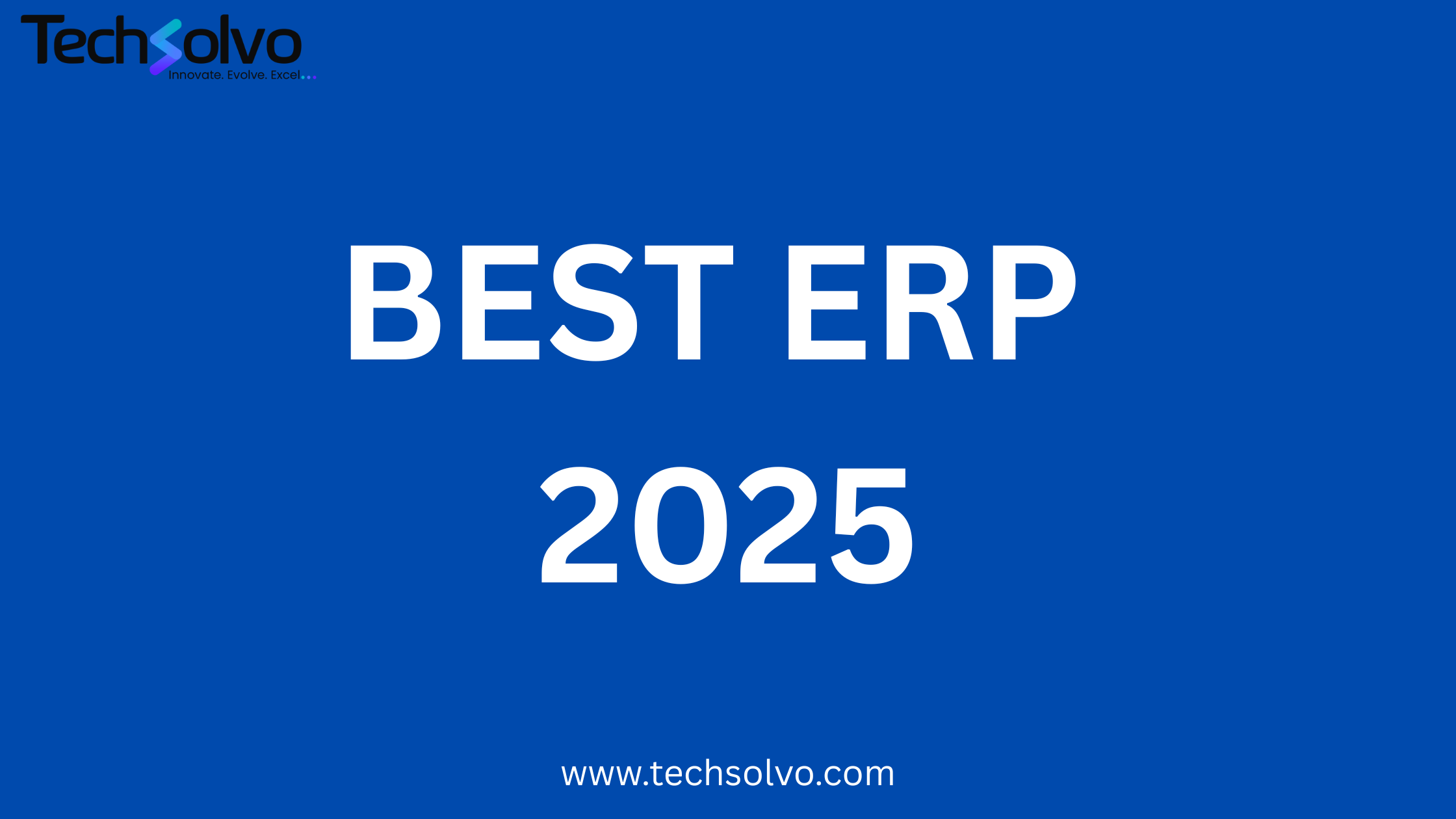
Why ERPNext is the Best ERP Software for Businesses in 2025 | Techsolvo
Discover why ERPNext is the top choice for modern businesses in 2025. Learn how Techsolvo…
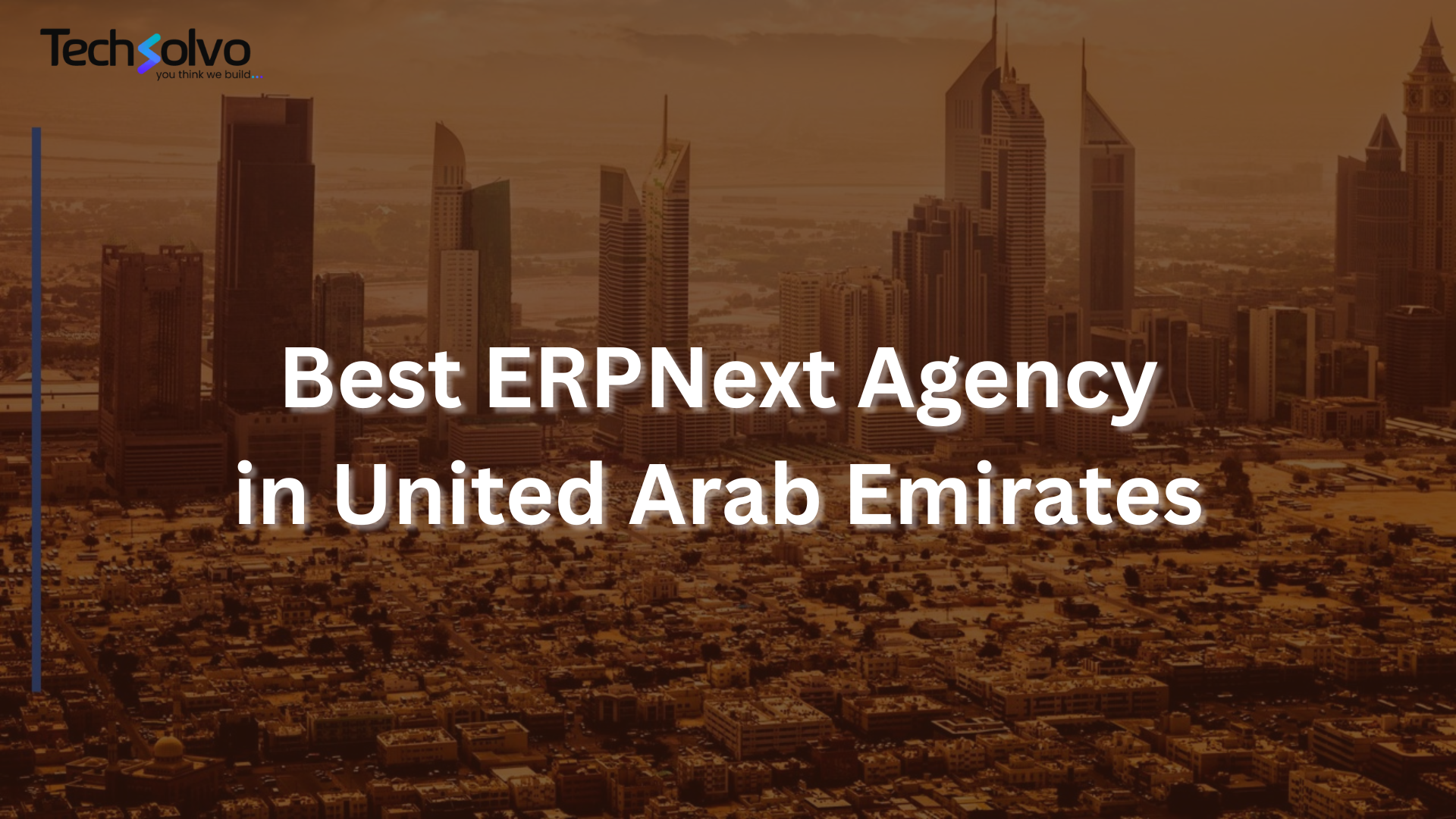
Why Techsolvo is the Best ERPNext Implementation Partner in UAE
Discover why Techsolvo is recognized as the best ERPNext agency in the UAE. Trusted by ma…
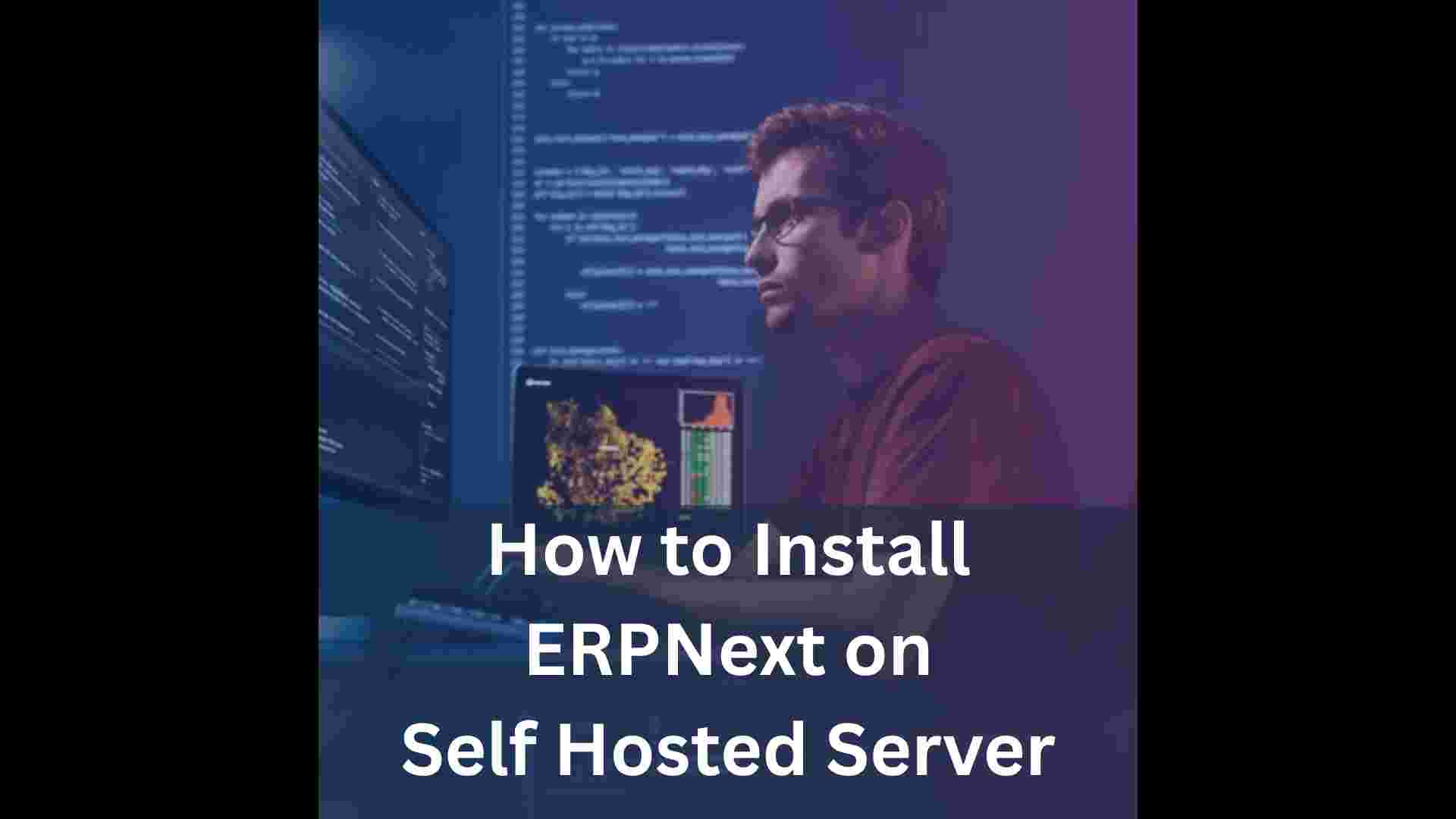
How We Self-Hosted ERPNext with Cloudflare Tunnel and Fixed Static Asset Issues
Learn how Techsolvo successfully deployed a self-hosted ERPNext instance using Cloudflare…